Evolving Six Sigma in the Industry 4.0: Embracing Multivariate Tools for a New Era
11 oct 2024
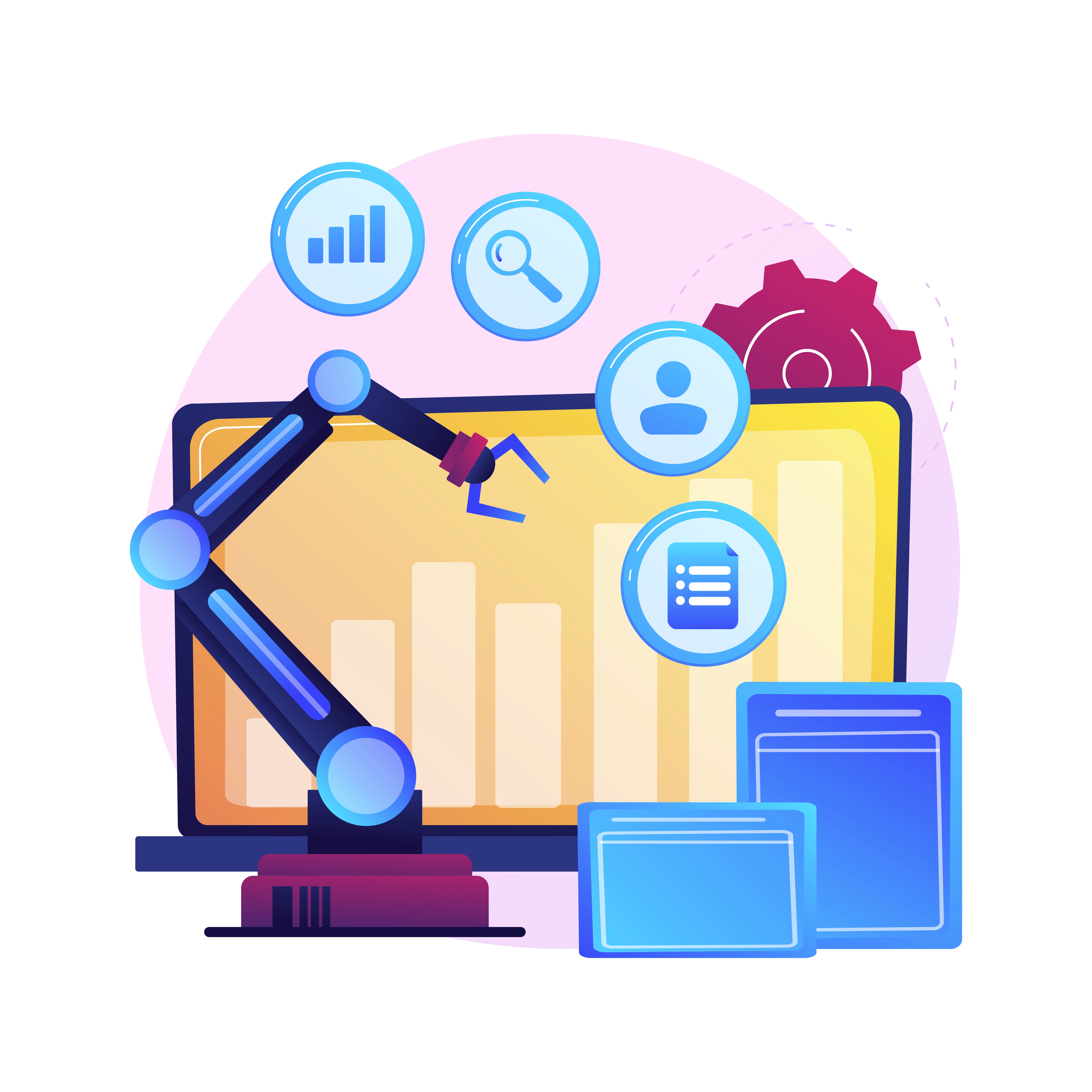
Industry 4.0 represents the fourth industrial revolution, characterized by the integration of cyber-physical systems, the Internet of Things (IoT), and Big Data analytics into manufacturing and production processes. This new paradigm has led to an exponential increase in the amount of data generated within industrial processes. As a result, many questions arise in this new context.
Is this true that Data Science, with its powerful machine learning tools, is the future in which we must bet to guarantee the survival of companies in the new Big Data environment of Industry 4.0?
Does it make sense to continue betting on the scientific method and statistical thinking with subject matter knowledge for understanding the root causes that cause problems, or all we need is exploiting the abundance of data generated in this new environment with powerful algorithms and computing facilities?
What are the challenges for Six Sigma to remain a successful improvement strategy in Industry 4.0?
The Role of Data Science in Industry 4.0
With the advent of Industry 4.0, Data Science has become a critical field, focusing on extracting meaningful insights from vast datasets using advanced computational techniques, including machine learning. It yields a tension between the rise of data-driven approaches, which often prioritize prediction and pattern recognition, and traditional statistical thinking, which emphasizes understanding causality and the underlying mechanisms of processes. Givent this, Six Sigma must remain effective and must incorporate both perspectives—leveraging the predictive power of Data Science while maintaining the rigorous, causal approach of traditional statistical methods.
Challenges for Traditional Six Sigma
Six Sigma has historically relied on a structured approach to process improvement, using statistical tools designed for environments where data was relatively scarce and easy to manage. However, in the context of Industry 4.0, these traditional tools may fall short due to the following challanges:
Data Abundance: The sheer volume of data generated by modern industrial processes can overwhelm traditional Six Sigma tools.
High-Dimensionality: Modern datasets often involve a large number of variables, leading to high-dimensional data spaces that require more sophisticated analysis methods.
Complex Interactions: Industry 4.0 processes often involve complex, nonlinear interactions between variables that are difficult to capture using traditional linear models.
The Need for Multivariate Analysis Tools
To address these challenges, there is a need for integrating of multivariate statistical techniques into the Six Sigma toolkit. In this sense, machine learning tools and and latent variable-based models are of interest.
Machine learning tools may play a critical role in the Measure and Analyze phases to find and interpret patterns and for predictive purposes.
On the other hand, latent variable-based models are statistical models specifically designed to analyze massive amounts of correlated data. The basic idea behind LVMs is that the number of underlying factors, (called latent variables), acting on a process is much smaller than the number of measurements taken on the system. The following are the most popular tools:
Principal Component Analysis (PCA): PCA is a dimensionality reduction technique that can simplify complex datasets by identifying the most important variables, or components, that explain the most variance in the data.
Partial Least Squares (PLS) Regression: PLS is a predictive modeling technique that can handle highly collinear data, making it suitable for the high-dimensional datasets typical of Industry 4.0.
In the Measure phase and at the beginning of the Analyze phase, PCA and PLS can be used for (multivariate) process monitoring, fault detection and diagnosis, typical tasks in multivariate statistical process control (MSPC). Traditional univariate SPC (taught in most of the Six Sigma training courses) should be enriched with MSPC. PLS can also be used for building predictive models (as machine learning tools). But the most remarkable characteristic of PLS models is that they not only model the relationship between X and Y (as classical linear regression and machine learning models do), but also provide models for both the X and Y spaces. This fact gives them very nice properties: uniqueness and causality in the reduced latent space (this is the only space within which the process has varied) no matter if the data come either from a design of experiments (DOE) or daily production process (historical/happenstance data). These properties make them suitable for process optimizaton in the Improve phase.
Conclusion: Evolving Six Sigma for Industry 4.0
Six Sigma must remain relevant and effective in the era of Industry 4.0, it must evolve. This evolution involves incorporating modern data science techniques while preserving the foundational principles of statistical thinking, such as causality and the scientific method. By embracing tools like PCA and PLS, Six Sigma can continue to be a powerful strategy for quality improvement and process excellence in the data-rich environment of Industry 4.0.
Ferrer, A. (2021). Multivariate six sigma: A key improvement strategy in industry 4.0. Quality Engineering, 33(4), 758-763.