Case study: application of multivariate statistical process control (MSPC)
29 oct 2024
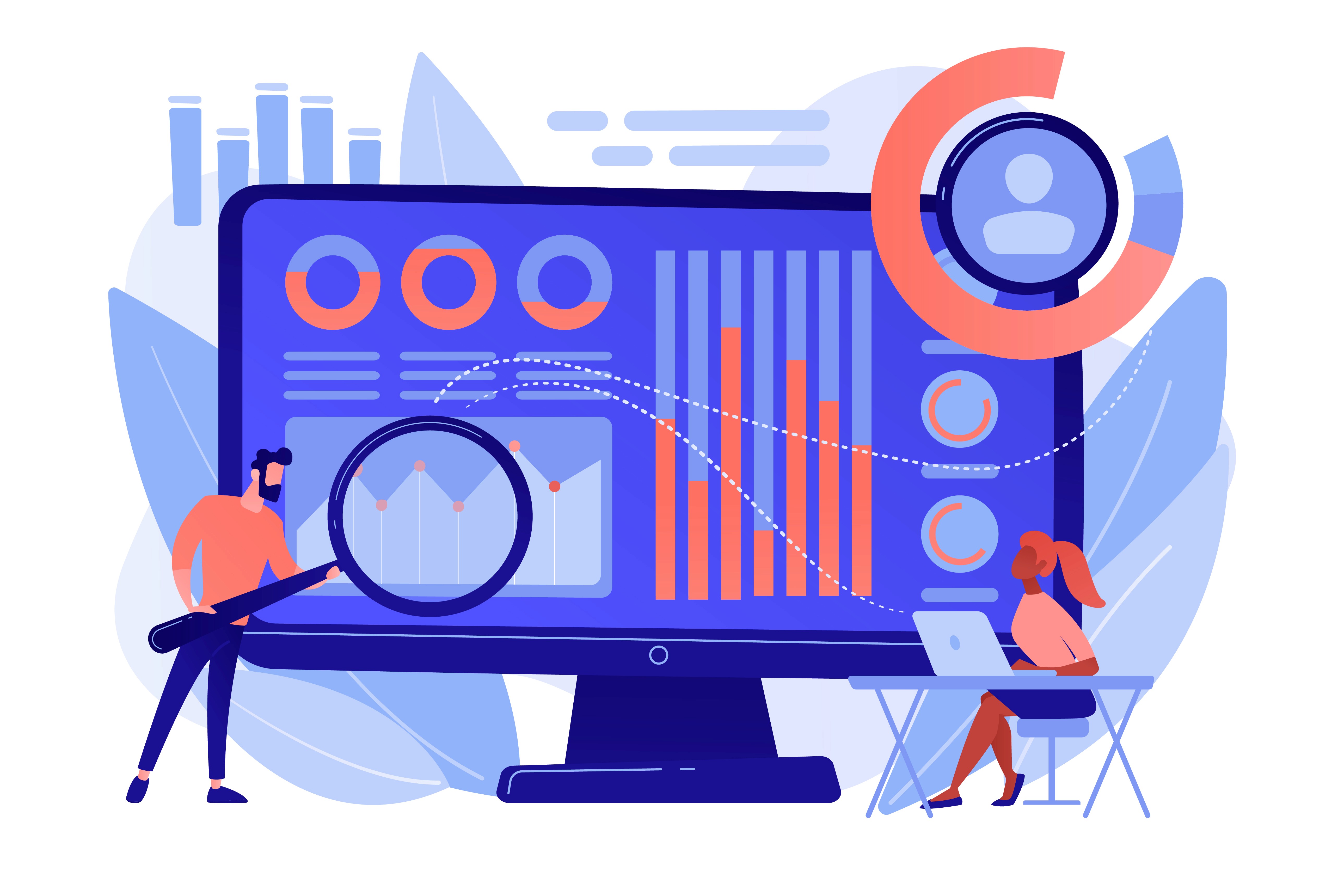
Context
In a manufacturing environment, it is essential to maintain consistent quality standards and to identify and address process deviations in a timely manner.
In a large-scale manufacturing environment, it is essential to guarantee consistent quality and to identify process deviations in a timely manner. It is often the case that traditional quality control methods, which are based on basic univariate or multivariate approaches, are inadequate in environments where data is generated by multiple sensors and machine operations. This is because such operations often result in the generation of large volumes of correlated, high-dimensional data, which is characterised by a large number of recorded variables. Conventional statistical process control (SPC) is not always effective in addressing issues such as autocorrelation between sensors or missing values in real-time data entry. This can lead to reduced monitoring effectiveness and delayed detection of faults that may impact product quality.
Problem
Let us suppose that a chemical manufacturer has been experiencing quality issues with a specific product line on an unplanned and intermittent basis. Despite applying traditional SPC control charting techniques, the company has found that variability in certain product parameters is often detected too late, resulting in increased rework costs and potential production interruptions. Furthermore, given the interdependencies between various process variables (e.g. temperature, pressure and production line speed), traditional SPC control charts have difficulty capturing underlying patterns and diagnosing the root causes of out-of-control situations.
Solution Using LSb-MSPC
To address these challenges, LSb-MSPC is implemented as follows:
Data Collection and Preprocessing:
Historical data is collected from a variety of sensors, including those on the machine itself and external elements that could potentially influence the process. The focus is on variables known to affect product quality, such as temperature, piston pressure, threader rotational speed, and room humidity. Any missing values are estimated using data imputation techniques compatible with latent structure models.
Building the LSb-MSPC Model:
Using PCA and PLS, high-dimensional sensor data is projected into a lower-dimensional latent space, where the essential patterns of the process are extracted. This is like summarising a complex text by extracting the key points without having to read the whole text. Thanks to these techniques, patterns in the data can be extracted in an efficient way.
Hotelling's T2 and SPE statistics are calculated, which capture both score-based process variations and those based on model residuals.Hotelling’s T2 and SPE statistics are computed, capturing both the score-based and residual-based variations of the process.
Monitoring in Two Phases:
Phase I: The LSb-MSPC model is developed using historical, in-control data, which establishes the baseline for normal process conditions.
Phase II: New real-time process data is compared with established control charts. Should any out-of-control signals be identified, diagnostic procedures will be initiated.
Fault Diagnosis and Root Cause Analysis:
When an out-of-control signal is identified, contribution charts assist in determining which original variables contribute the most to the deviations. This enables the rapid identification of specific process variables, such as an unexpected increase in piston pressure or temperature spikes, that may have caused the observed quality issues.
Industrial applications
In a manufacturing context, LSb-MSPC provides an effective solution for managing high-dimensional data (many variables) and diagnosing quality problems in complex processes. By implementing LSb-MSPC, manufacturers could:
Proactive quality control: The manufacturer can detect quality deviations much earlier than with traditional SPC, reducing rework costs and improving consistency.
Data complexity management: LSb-MSPC manages dependencies between variables and missing data, enabling more accurate real-time monitoring.
Rapid Troubleshooting: Contribution graphs quickly identify which process variables are causing failures, enabling targeted troubleshooting and reduced downtime.
Outcome
By implementing LSb-MSPC, the manufacturer was able to achieve a 22% reduction in rework costs and a notable decrease in production interruptions. Furthermore, the early fault detection capabilities of the latent structure model empowered operators to take corrective action with greater efficiency, reducing outages, enhancing process comprehension, and stabilising overall operations.
Ferrer, A. (2014). Latent structures-based multivariate statistical process control: A paradigm shift. Quality Engineering, 26(1), 72-91.